COLLABORATE ON OUR
Scientific Experiments
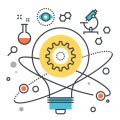
The Virtual Lab grants access to the experiments performed in all scientific domains of the PrimeWater project: remote sensing, process-based modeling, and data-driven modeling. The Virtual Lab describes in detail the data, equipment, and experimental procedures followed in each experiment to enhance transparency and secure the reproducibility of results. Nonetheless, the Virtual Lab is not a mere repository of experiments; it welcomes and embraces collaborative research efforts across organizational boundaries. The Virtual Lab facilitates research groups to exchange knowledge in a collaborative framework that will ultimately advance remote sensing and hydro-ecological modeling.